Is There Really a Need for Data Cleansing?
The importance of clean data cannot be understated. Unfortunately, cleaning data is difficult to get on the agenda and to be allocated budget. Experian reports that on average companies data is only around 75% clean. This is costing significantly both in reputation and money. For example, in this day of strict privacy, having dispersed sources of data where the user may have consented at one point but not another, may lead to breaches of Privacy Laws.
The ROI on putting in place some data cleansing functions will be positive if the right steps are taken. But, it is only when senior executives call for “trusted” data that the problem can be seriously addressed.
What Types of Data Problems are There?
There are 5 types of data problems:
- Incomplete Data.
- Duplicate Data
- Inaccurate data.
- Inconsistent data
- Outdated data
How Are Data Corruption Issues Prevented?
Solving each type of data problem needs a different approach. But let’s look at prevention.
One of the best approaches is having a Single Source of Truth (SSOT) but this is an architectural solution that can only be addressed by a large IT Department change in strategy. This ensures all – well as many as possible – use the same source of data for common data such as client data, address data and contact information. The data duplication, inaccurate data, inconsistent and outdated data cleansing still need to be done but it will be in one place and less of it. In the meantime, the following can be done.
Another important tool in prevention is having standard approaches across all systems to ensure formats, rules and metadata are the same for the same type of data.
Reporting on Data Cleansing Problems
You will need to do data profiling providing adequate analysis reporting to identify data problems enabling measurement, visualisation and data profiling. Managers need to understand where the problems are located. Once problems are identified steps can be taken to fix the problems
How Are Data Cleansing Problems Fixed?
Whether it is incomplete, duplicate, inaccurate, inconsistent or outdata data problems that need to be tackled, there are many problems to assist in cleaning.
For data duplication, this is perhaps the easiest because the tools allow selection of some fields to compare and identify duplicates. Then making a decision on which of the duplicates is to be removed or merged with other data. This problem will require some manual intervention and oversight.
With outdated data, in some cases purging data after a certain date may be appropriate but usually not feasible. Sometimes, these can be tackled by going to more up-to-date sources on the web and running updates.
Inaccurate, incomplete and inconsistent data all need to be identified first. Then the decision can be made on the approach. One approach is to use externally sourced data, to update and improve the data. The other is to examine the data and apply a series of standards edits e.g. remove spaces and padding that is not needed, fix spelling mistakes, hot-deck (copy same data from other valid sources), fixing capitalization. Once problems are identified manual intervention can allow you to do bulk updates.
Magic Quadrant for Data Quality Solutions
The solutions for cleansing data quality are improving. The Gartner Magic Quadrant for Data Quality shows the top programs.
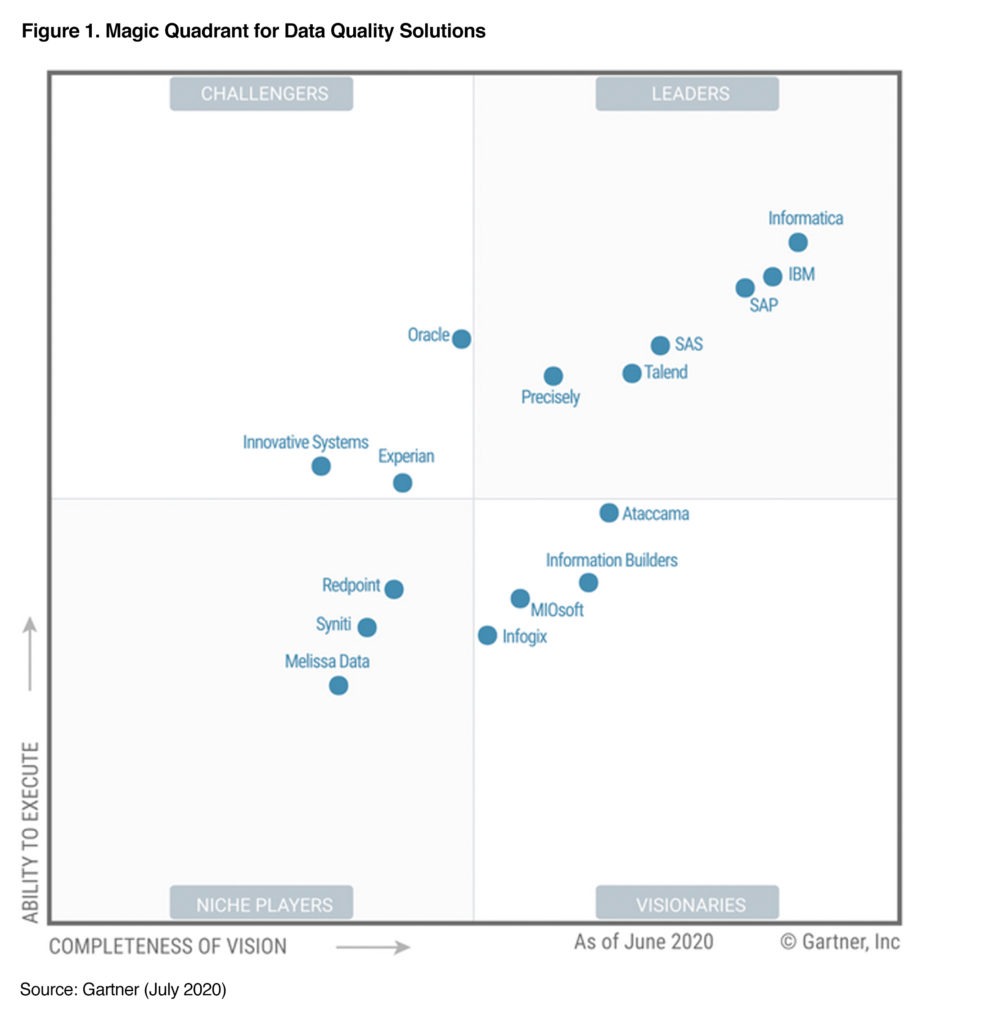
Data Cleansing Software List
There is now significant software and processes to help companies clean up their data including the following: